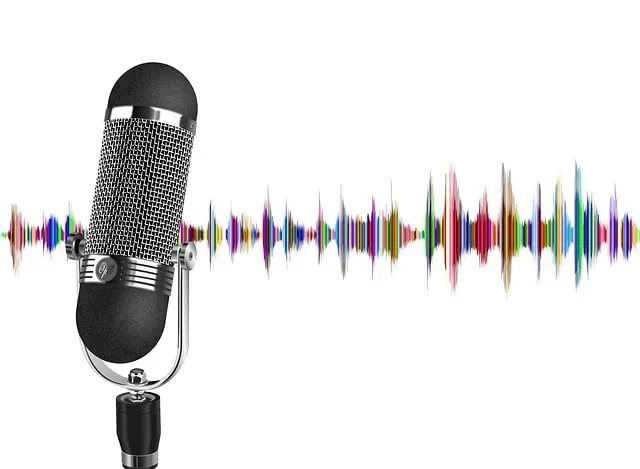
How To Make a Speech Recognition Algorithm
In this post, we’re going to make a simple speech recognition algorithm using Tensorflow machine learning library. Furthermore, the following project entails making a classification algorithm, which will classify between 30 different sounds.
Essentially, we’ll need to preprocess our audio files and convert them into images or spectrograms. Therefore, we’ll be able to use convolutional neural network for this problem.
We’re also going to implement a couple of data augmentation techniques for audio files, including adding background noise.
About dataset
The dataset has a total of 30 folders, 1 for each class. It also contains 1 extra folder, which holds the background noise audio files. Furthermore, each folder has audio files of different speakers saying the same word.
Additionally, we’ll not be using the background noise audio files as its own class. However, we will randomly add it to other audio files.
The words, which our speech recognition algorithm will classify are:
- yes
- no
- up
- down
- left
- right
- on
- off
- stop
- go
- zero
- one
- two
- three
- four
- five
- six
- seven
- eight
- nine
- bed
- bird
- cat
- dog
- happy
- house
- Marvin
- Sheila
- tree
- wow
Coding speech recognition algorithm
As we already mentioned, we’re going to make this with Tensorflow. However, it is just one of the necessary libraries we’ll need for this project. So, first things first, let’s import all these libraries and functions.
import os
from glob import glob
from pyunpack import Archive
import pandas as pd
import numpy as np
import tensorflow as tf
from keras import Input, layers
from sklearn.preprocessing import StandardScaler
import math
import librosa
We’ve already encountered most of these libraries in the previous machine learning examples. However, since this is the first example where we’re dealing with audio, we’ll need librosa library. To explain, it’s a library for processing audio signals.
Next step, before we can do anything, we need to extract and position our dataset, which came in a .zip format. We’re also going to set seed for randomizing functions, so we’ll be able to reproduce the results.
root = os.path.dirname(__file__)
seed = 42
tf.random.set_seed(seed)
np.random.seed(seed)
if not os.path.exists(os.path.join(root, 'working', 'train')):
os.makedirs(os.path.join(root, 'working', 'train'))
os.mkdir(os.path.join(root, 'tensorflow-speech-recognition-challenge'))
Archive(
root + '/tensorflow-speech-recognition-challenge.zip'
).extractall(root + '/tensorflow-speech-recognition-challenge')
Archive(root + '/tensorflow-speech-recognition-challenge/train.7z').extractall(root + '/working')
train_path = os.path.join(root, 'working', 'train', 'audio')
Next, we’ll define all the functions we’ll be using for processing each audio file.
def pad_audio(samples, L):
if len(samples) >= L:
return samples
else:
return np.pad(samples, pad_width=(L-len(samples), 0), mode='constant', constant_values=(0, 0))
def chop_audio(samples, L=16000):
while True:
beg = np.random.randint(0, len(samples) - L)
yield samples[beg: beg + L]
def choose_background_generator(sound, backgrounds, max_alpha=0.7):
if backgrounds is None:
return sound
gen = backgrounds[np.random.randint(len(backgrounds))]
background = next(gen) * np.random.uniform(0, max_alpha)
augmented_data = sound + background
augmented_data = augmented_data.astype(type(sound[0]))
return augmented_data
def random_shift(sound, shift_max=0.2, sampling_rate=16000):
shift = np.random.randint(sampling_rate * shift_max)
out = np.roll(sound, shift)
if shift > 0:
out[:shift] = 0
else:
out[shift:] = 0
return out
def random_change_pitch(x, sr=16000):
pitch_factor = np.random.randint(1, 4)
out = librosa.effects.pitch_shift(y=x, sr=sr, n_steps=pitch_factor)
return out
def random_speed_up(x):
where = ['start', 'end'][np.random.randint(0, 1)]
speed_factor = np.random.uniform(0, 0.5)
up = librosa.effects.time_stretch(x, rate=(1 + speed_factor))
up_len = up.shape[0]
if where == 'end':
up = np.concatenate((up, np.zeros((x.shape[0] - up_len))))
else:
up = np.concatenate((np.zeros((x.shape[0] - up_len)), up))
return up
In the following step, we’re going to define functions that will load in the audio files and preprocess them.
def get_image_list(train_audio_path):
classes = os.listdir(train_audio_path)
classes = [c for c in classes if c != '_background_noise_']
index = [i for i, j in enumerate(classes)]
out = []
labels = []
for i, c in zip(index, classes):
files = [f for f in os.listdir(os.path.join(train_audio_path, c)) if f.endswith('.wav')]
files = [os.path.join(train_audio_path, c, f) for f in files]
out.append(files)
labels.append(np.full(len(files), fill_value=i))
return out, labels, dict(zip(classes, index))
def split_train_test_stratified_shuffle(images, labels, train_size=0.9):
classes_size = [len(x) for x in images]
classes_vector = [np.arange(x) for x in classes_size]
total = np.sum(classes_size)
total_train = [int(train_size * total * x) for x in classes_size / total]
train_index = [np.random.choice(x, y, replace=False) for x, y in zip(classes_size, total_train)]
val_index = [np.setdiff1d(i, j) for i, j in zip(classes_vector, train_index)]
train_set = [np.array(x)[idx] for x, idx in zip(images, train_index)]
val_set = [np.array(x)[idx] for x, idx in zip(images, val_index)]
train_labels = [np.array(x)[idx] for x, idx in zip(labels, train_index)]
val_labels = [np.array(x)[idx] for x, idx in zip(labels, val_index)]
train_set = np.array([el for array in train_set for el in array])
val_set = np.array([el for array in val_set for el in array])
train_labels = np.array([el for array in train_labels for el in array])
val_labels = np.array([el for array in val_labels for el in array])
train_shuffle = np.random.permutation(len(train_set))
val_shuffle = np.random.permutation(len(val_set))
train_set = train_set[train_shuffle]
val_set = val_set[val_shuffle]
train_labels = train_labels[train_shuffle]
val_labels = val_labels[val_shuffle]
return train_set, train_labels, val_set, val_labels
def preprocess_data(file, background_generator, target_sr=16000, n_mfcc=40, threshold=0.7):
x, sr = librosa.load(file, sr=target_sr)
x = pad_audio(x, sr)
if np.random.uniform(0, 1) > threshold:
x = choose_background_generator(x, background_generator)
if np.random.uniform(0, 1) > threshold:
x = random_shift(x)
if np.random.uniform(0, 1) > threshold:
x = random_change_pitch(x)
if np.random.uniform(0, 1) > threshold:
x = random_speed_up(x)
mfccs = librosa.feature.mfcc(y=x, sr=sr, n_mfcc=n_mfcc)
mfccs = np.moveaxis(mfccs, 1, 0)
scaler = StandardScaler()
mfccs_scaled = scaler.fit_transform(mfccs)
return mfccs_scaled.reshape(mfccs_scaled.shape[0], mfccs_scaled.shape[1], 1)
class DataGenerator(tf.keras.utils.Sequence):
def __init__(self, x_set, y_set, batch_size, background_generator):
self.x, self.y = x_set, y_set
self.batch_size = batch_size
self.background_generator = background_generator
def __len__(self):
return math.ceil(len(self.x) / self.batch_size)
def __getitem__(self, idx):
idx_from = idx * self.batch_size
idx_to = (idx + 1) * self.batch_size
batch_x = self.x[idx_from:idx_to]
batch_y = self.y[idx_from:idx_to]
x = [preprocess_data(elem, self.background_generator) for elem in batch_x]
y = batch_y
return np.array(x), np.array(y)
Next, we need to define the function that will create our machine learning model.
def build_model(n_classes, input_shape):
model_input = Input(shape=input_shape)
x = layers.Conv2D(32, (3, 3), padding='same', activation='relu')(model_input)
x = layers.MaxPool2D((2, 2))(x)
x = layers.Conv2D(64, (3, 3), padding='same', activation='relu')(x)
x = layers.MaxPool2D((2, 2))(x)
x = layers.Conv2D(128, (3, 3), padding='same', activation='relu')(x)
x = layers.MaxPool2D((2, 2))(x)
x = layers.Conv2D(256, (3, 3), padding='same', activation='relu')(x)
x = layers.MaxPool2D((2, 2))(x)
x = layers.Dropout(0.25)(x)
x = layers.Flatten()(x)
x = layers.Dense(128, activation='relu')(x)
x = layers.Dropout(0.5)(x)
model_output = layers.Dense(n_classes, activation='softmax')(x)
return tf.keras.Model(model_input, model_output)
In the following section, we’re going to start and use all the functions we defined so far. Furthermore, this includes preparing dataset for inputing it into the model and setting hyperparameters.
bg_files = glob(os.path.join(train_path, '_background_noise_', '*.wav'))
bg_files = [librosa.load(elem, sr=16000)[0] for elem in bg_files]
background_generator = [chop_audio(x) for x in bg_files]
images, labels, classes_map = get_image_list(train_path)
train_set, train_labels, val_set, val_labels = split_train_test_stratified_shuffle(images, labels)
train_datagen = DataGenerator(train_set, train_labels, 40, background_generator)
val_datagen = DataGenerator(val_set, val_labels, 40, None)
rows = 32
columns = 40
batch_size = 100
epochs = 50
base_path = os.path.join(root, 'working', 'models')
if not os.path.exists(base_path):
os.makedirs(base_path)
train_size = train_set.shape[0]
val_size = val_set.shape[0]
steps_per_epoch = train_size // batch_size
lr = 1e-3
Now, we’re ready for compiling and training the model. We’re also going to setup a few callback functions, including early stopping, reducing learning rate, and saving weights.
checkpoint_path = os.path.join(base_path, 'cp-{epoch:04d}.ckpt')
checkpoint_callback = tf.keras.callbacks.ModelCheckpoint(
filepath=checkpoint_path,
save_best_only=True,
save_weights_only=True,
monitor='val_loss',
mode='min',
verbose=1
)
reduce_lr_callback = tf.keras.callbacks.ReduceLROnPlateau(
monitor='val_loss',
factor=0.2,
patience=3,
min_lr=1e-5,
verbose=1
)
earlystopping_callback = tf.keras.callbacks.EarlyStopping(
monitor='val_loss',
min_delta=1e-3,
patience=5,
verbose=1
)
optimizer = tf.keras.optimizers.Adam(learning_rate=lr)
loss_fn = tf.keras.losses.SparseCategoricalCrossentropy()
acc_metric = tf.keras.metrics.SparseCategoricalAccuracy()
model = build_model(len(classes_map), (rows, columns, 1))
model.compile(
optimizer=optimizer,
loss=loss_fn,
metrics=[acc_metric]
)
model.load_weights(os.path.join(base_path, 'cp-0024.ckpt'))
print(model.summary())
history = model.fit(
train_datagen,
steps_per_epoch=steps_per_epoch,
epochs=epochs,
validation_data=val_datagen,
validation_steps=val_size//batch_size,
callbacks=[earlystopping_callback, reduce_lr_callback, checkpoint_callback]
)
In order to see the final results, we need to evaluate the models performance using test dataset. Furthermore, test audio files are zipped into a separate archive. Therefore, we’ll need to extract it and preprocess it in a similar way as we did with training dataset.
test_path = os.path.join(root, 'working', 'test')
if not os.path.exists(test_path):
os.makedirs(test_path)
Archive(root + '/tensorflow-speech-recognition-challenge/test.7z').extractall(root + '/working')
test_data, test_labels, _ = get_image_list(test_path)
test_data = test_data[0]
test_labels = test_labels[0]
test_datagen = DataGenerator(test_data, test_labels, batch_size, None)
test_size = len(test_data)
test_steps = np.ceil(test_size / (batch_size))
y_pred = model.predict_generator(test_datagen, steps=test_steps, verbose=1)
y_labs = np.argmax(y_pred, axis=1)
inv_map = {v: k for k, v in classes_map.items()}
print(inv_map)
test_audio_sample = test_data[16]
print(test_audio_sample)
x,sr = librosa.load(test_audio_sample, sr = 16000)
print(inv_map[y_labs[16]])
Preceding image shows the final results for a randomly selected sample from test dataset. First line shows the audio file path and second line is the class it belongs to.
Conclusion
To conclude, we managed to create a simple speech recognition algorithm, which classifies audio files into the word classes that are spoken in them.
I hope this tutorial helped you gain a better understanding of how audio processing in machine learning works and how we can utilize it.