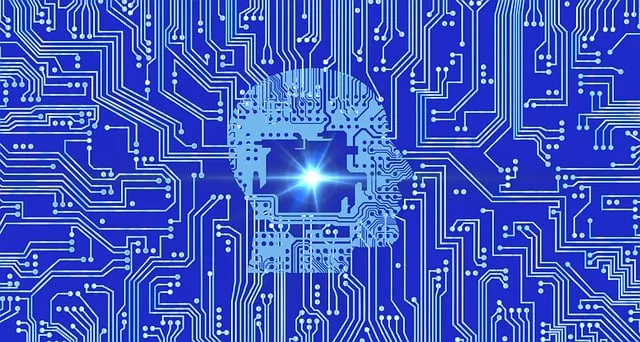
Applications of Generative Adversarial Networks
Applications of Generative Adversarial Networks (GANs) have revolutionized the fields of artificial intelligence and machine learning. Thus paving the way for groundbreaking implementations.
As a class of deep learning models, they possess the unique ability to generate and manipulate data. Therefore, giving rise to numerous practical applications that have transformative potential.
Furthermore, their development made it possible to create realistic images, sounds, and other forms of data. This has led to innovative applications in fields such as art, entertainment, and even medicine.
By exploring these real-world applications, we can gain a deeper understanding of the power and versatility of GANs. Which will also give us a reason to appreciate the challenges and opportunities that lie ahead.
Applications of Generative Adversarial Networks for Image Synthesis and Manipulation
Artistic Style Transfer: Fusing Creativity and Technology
One of the most captivating applications of Generative Adversarial Networks is the creation of artistic style transfers.
By combining the content of one image with the stylistic elements of another, they enable the generation of visually striking, novel images.
We can use them to create mesmerizing artwork and expand the boundaries of digital art.
Image Inpainting: Restoring and Enhancing Visual Data
They have also demonstrated great potential in image inpainting. To clarify, that’s the process of filling in missing or corrupted parts of images with plausible content.
Additionally, by learning the underlying structure and patterns of an image, they can seamlessly reconstruct missing information. Thus, enabling the restoration of damaged photographs or the removal of unwanted objects from images.
This application has broad utility across various fields, including digital forensics, media production, and art conservation.
Face Generation and Aging: Unlocking New Possibilities in Entertainment and Beyond
They can also generate realistic human faces, leading to applications in entertainment, gaming, and virtual reality.
For example, they can predict age progression, simulating how an individual’s face might change over time.
This capability has numerous practical uses, such as aiding in missing person investigations and creating age-progressed images for identification purposes.
Applications of Generative Adversarial Networks for Data Augmentation and Generation
Text-to-Image Synthesis: Bringing Words to Life
GANs have enabled the generation of images from textual descriptions, a process known as text-to-image synthesis.
Furthermore, this application has significant implications for various industries, including advertising, entertainment, and e-commerce.
For instance, they can generate product images based on textual descriptions. Thus, creating unique and engaging visuals for marketing purposes. Even more, the can transform a script into a sequence of images for storyboard creation.
Audio Synthesis: Crafting Realistic Soundscapes
Generative Adversarial Networks have been applied to audio synthesis as well, enabling the generation of realistic sound samples for various applications.
In addition, this can be particularly useful in the entertainment industry for creating immersive soundscapes in films or video games.
Additionally, they can also generate synthetic speech or music, allowing for novel approaches to voice synthesis and music composition.
Generating Synthetic Datasets: Enhancing Data Availability for Machine Learning
GANs can generate synthetic datasets, which are particularly useful when real-world data is scarce, expensive, or privacy-sensitive.
Furthermore, we can use synthetic data to train machine learning models, improving their performance and robustness.
Additionally, this application is valuable in fields such as healthcare, finance, and autonomous systems. Basically, in domains, where access to large quantities of real data can be challenging or restricted.
Applications of Generative Adversarial Networks for Anomaly Detection and Cybersecurity
Identifying Unusual Patterns for Fraud Detection and Network Security
GANs were employed in anomaly detection, identifying unusual patterns in data for applications such as fraud detection and network security.
Furthermore, by learning the underlying structure of typical data, they can detect deviations from the norm. Thus, alerting security teams to potential threats or fraudulent activities.
This capability is essential for industries that handle sensitive information or require robust security measures, such as finance and healthcare.
Generating Adversarial Examples for Robustness Testing of AI Systems
GANs can generate slightly modified examples, which can be input data designed to mislead machine learning models.
Furthermore, by testing AI systems with these examples, developers can identify vulnerabilities and improve the robustness of their models.
Additionally, this application is crucial for ensuring the reliability and safety of AI systems across various domains, from autonomous vehicles to facial recognition systems.
Applications of Generative Adversarial Networks for Drug Discovery and Molecular Design
De Novo Molecular Generation: Designing Novel Molecules with Desired Properties
GANs have shown promise in de novo molecular generation, the design of novel molecules with desired properties.
By learning the structure and characteristics of known molecules, they can generate new molecules with specific target properties.
For example, binding affinity or drug-like qualities. This application has the potential to accelerate drug discovery and reduce costs. Thus, enabling faster development of novel therapeutics.
Drug Repurposing: Identifying New Therapeutic Uses for Existing Drugs
Generative Adversarial Networks can also aid in drug repurposing, the identification of new therapeutic uses for existing drugs.
By generating novel molecular structures and predicting their interactions with biological targets, they can help researchers uncover previously unknown applications for approved medications.
Therefore, potentially reducing the time and expense associated with traditional drug development processes.
Conclusion
In this post, we have explored the diverse and transformative real-world applications of Generative Adversarial Networks.
From image synthesis and manipulation to data augmentation and generation, GANs have demonstrated their potential to revolutionize various industries.
Their applications in anomaly detection and cybersecurity underscore their importance in addressing critical security concerns, while their role in drug discovery and molecular design highlights their potential to accelerate the development of life-changing therapeutics.
The Importance of Continued Research and Development for Generative Adversarial Networks Applications
Understanding the real-world applications of GANs highlights the significance of continued research and development in this area.
As they improve, they will become increasingly capable of addressing more complex challenges and generating even more realistic and useful data.
Furthermore, investing in GAN research is essential for ensuring that this powerful technology reaches its full potential and continues to benefit various industries.
I also hope this article helped you gain a better understanding of how we can utilize generative adversarial networks in practice and perhaps even inspire you to learn even more.